Jeff Hawkins is the founder of the Redwood Center for Theoretical Neuroscience, a nonprofit scientific research facility, in 2002, and Numenta, a machine intelligence company, in 2005. Hawkins is currently Chief Scientist at Numenta, where he leads a team in efforts to reverse-engineer the neocortex and enable machine intelligence technology based on brain theory. Hawkins is the author of a recently published book “A Thousand Brains: A New Theory of Intelligence” which explains his memory-prediction framework theory of the brain. He and his team discovered that the brain uses maplike structures to build a model of the world – not just one model, but tens of thousands of models of everything we know. This discovery allows Hawkins to answer important questions about how we perceive the world, why we have a sense of self, and the origin of high-level thought.
His idea originated from a proposal of Vernon Benjamin Mountcastle, an American neurophysiologist and Professor Emeritus of Neuroscience at Johns Hopkins University. He suggested that the reason the brain regions look similar is that they are all doing the same thing. What makes them different is not their intrinsic function but what they are connected to. If you connect a cortical region to the eyes, you get vision; if you connect the same cortical region to ears, you get hearing; and if you connect regions to other regions, you get higher thought, such as language. Prof. Mountcastle points out that if we can discover the basic function of any part of the neocortex, we will understand how the entire thing works. In short, Mountcastle proposed that all the things we associate with intelligence, which on the surface appear to be different, are in reality, manifestations of the same underlying cortical algorithm.
Jeff Hawkins and his team further investigated the importance of the neocortex and how the brain works. In summary, they proposed that the neocortex is the area of the brain that learns a predictive model of the world through so-called cortical columns. There are around 150,000 cortical columns in a human neocortex and each of the cortical columns learns models of objects. The columns do this using the same basic method that the old brain (including the brain stem, medulla, pons, reticular formation, thalamus, cerebellum, amygdala, hypothalamus, and hippocampus that regulates basic survival functions, such as breathing, moving, resting, feeding, emotions, and memory) uses to learn models of environments. Therefore, each cortical column has a set of cells equivalent to grid cells, another set equivalent to place cells, and another set equivalent to head direction cells, all of which were first discovered in parts of the old brain. The predictions of each object actually occur inside neurons. Specifically, dendrite spikes of the neurons are predictions, but these predictions are not necessarily sent along the cell’s axon to all other neurons, which explains why we are unaware of most of them (e.g. if we are doing a task, we might ignore all sounds and images around us until something unexpected happens, however, the brain has to perceive all sensory information around us).
The main important feature of the cortical column is reference frames. Each cortical column must know the location of its input relative to the object being sensed. Thus, a cortical column requires a reference frame that is fixed to the object (imagine something like a coordinate system on the map).
In short, this is a simplified explanation of their discoveries that are still not complete because figuring how the brain works is hard, but it is a necessary step to creating intelligent machines. At the moment deep learning networks perform well, but only on very specific tasks, and we are still far away from solving the knowledge representation problem – how can we represent information about the world in a form that a computer system can utilize to solve complex tasks. Furthermore, currently, deep learning networks avoid this problem completely, relying on statistics and lots of data instead. However, the maps in the brain represent knowledge about the objects we interact with and we would require to address this question if we want to transfer our knowledge to machines.
According to Jeff Hawkings, the most important attributes of Artificial General Intelligence (AGI) which is the hypothetical ability of an intelligent agent to understand and learn any intellectual task that a human being can, are the following:
- AGI needs to be learning continuously.
- The learning happens via movement (not necessarily physical, e.g., we navigate through our apps, but this is a crucial part if we want to learn information about the world)
- The AGI needs to consist of many models that would provide flexibility and that you could integrate multiple sensors. Similarly, in the brain the system ‘votes’ on what object they are sensing – therefore, we could apply the same logic to the AGI that would get the multiple information from different sources and decided on the importance by voting.
- Finally, it is important that AGI would be using reference frames to store knowledge because this is a backbone of knowledge. Currently, deep learning networks lack a sense of location and, therefore, can’t learn the structure of the world.
If you would like to know more details about the theory, you could read the following paper https://www.frontiersin.org/articles/10.3389/fncir.2018.00121/full or his book. If you prefer a podcast, we also shared an interesting one with you below!
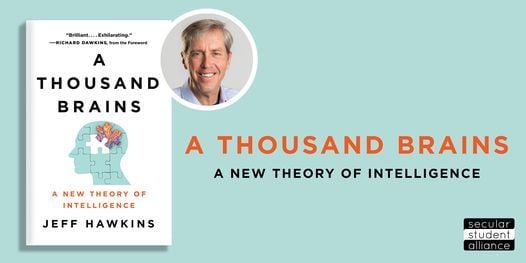