Claudio Pinhanez: Manager of the Conversational Intelligence Research group, IBM Research Brazil
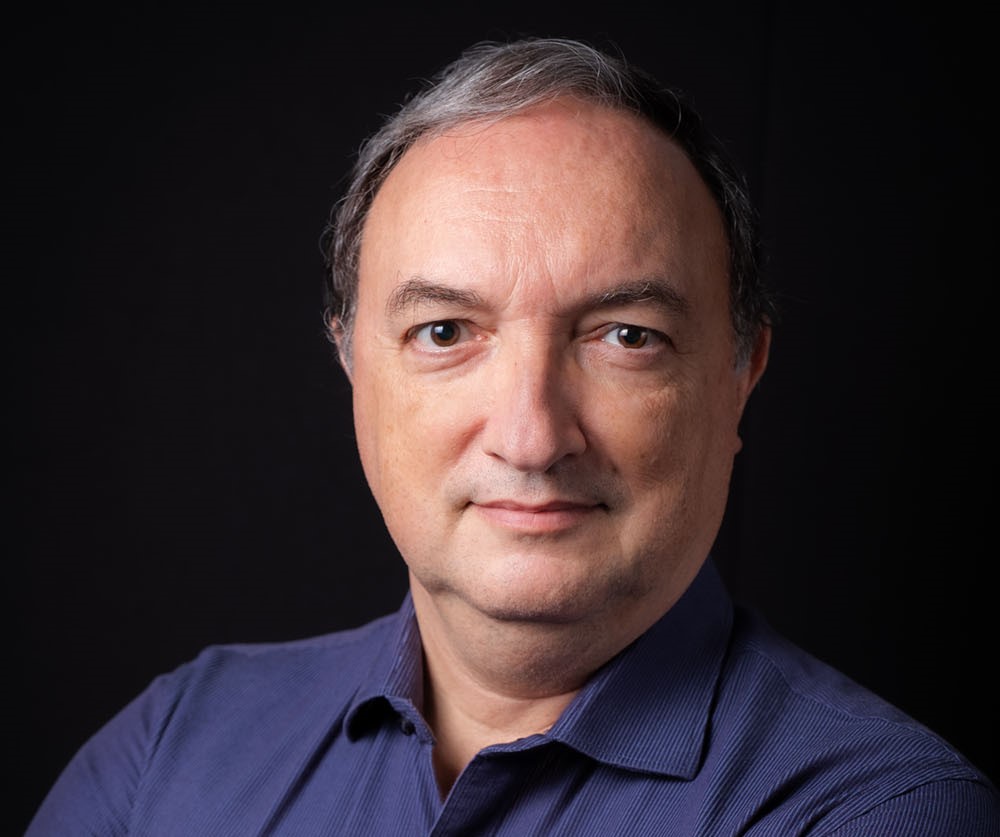
Dr. Claudio Pinhanez leads a 5-member group of Ph.Ds, software engineers, and students focused on advancing research and innovation in conversational systems, artificial intelligence, machine teaching, social computing, ubiquitous computing, and human-computer interfaces.
“Claudio got his Ph.D. in 1999 from the MIT Media Laboratory where he conducted multidisciplinary research on computer vision, interactive spaces, temporal algebras, and interactive art and theater. In his works, he created the precursors of many innovative camera-based interaction systems which were brought to the market 15 years later in devices such as the Kinect. He has also created the first Internet blog in 1994. After the Ph.D. Claudio was hired as a researcher at the T.J. Watson laboratory of IBM Research in New York where he worked from 1999 to 2008. There he invented the Everywhere Interactive Display, a revolutionary steerable projector-camera system that allowed on-demand interaction with any surface in an environment. This work was internationally recognized by a string of world-class publications, best paper awards, patents, and the 2003 Most Promising Scientist award from HENAAC (Hispanic Engineers National Achievement Awards Conference). In 2005 he started research in service science, a new scientific discipline focused on the service industries, and helped IBM lead the efforts to establish it internationally. In 2008 he was sent by IBM to Brazil to study the feasibility of a new IBM laboratory in Brazil. After 2 years of intensive conversations with the Brazilian government, academia, and industry, the IBM Research Brazil laboratory was founded in June of 2010. Since the foundation of the laboratory Claudio has worked in all aspects of the management of research and innovation groups, including human resources hiring and management, funding and finances, grant proposals, strategy, government contracts and incentives, operations, intellectual property, business development, and university relations. He has also built the largest service science research group in Brazil and now is leading the development of large-scale social media analytics systems in Portuguese such as the IBM FAMA used during the FIFA World Cup 2014.”
Below is a transcript of the Q&A session
Do you think there are differences between how children and adults perceive chatboxes, for instance, the expectations in terms of errors or how they are supposed to talk to you? We all know those examples when you type in a question and a chatbox completely misunderstands you and then people still try to get through to customer service to talk to a human being.
Yes, that’s an interesting question. When we are talking about children, we have to think about age. If you think about kids of four to eight, it is amazing how they use chatbox, they can learn any language they want at that time. They learn how to interact in very different ways, if you see a kid of that age interacting, they learn how to extract what they want as they do with any other adult around them. It is just amazing. But I think there is something interesting happening there, which is as speech recognition is introduced into interfaces, especially, Google search, YouTube, that enabled kids to do tasks like finding what they want on the Internet at a very young age, before they are literate, before they learn how to write. I have not seen any study, but I have seen some young kids interact, it is amazing how they do search. They learn how to say things and find things because they can adapt the language. This exhibit was targeted at kids who can write, but at the same time it is nice when you have this age group, nine to twelve. Seymour Papert, whom someone of you may know, says that it is the magic time with kids, kids can learn anything between nine and twelve. After that they get too afraid of looking stupid as they are becoming teenagers, but nine to twelve, it is magic. The behaviour of kids with that system vs. adults is quite different. So, the answer to the question is yes, but it is also more complicated because it is not children as a big thing, there are different categories and cognitive abilities within this group. It is amazing, especially with speech what is happening.
A rather broad question, what is your intuition in terms of closing the gap or improving the systems that produce fluent language, but have a rather limited understanding of human language? What does one need to work on for improving these systems and making them to understand more?
Well, first we have to recognize how much progress we have made. Anything like Siri and Alexa, if you asked me then years ago whether we were going to get where we are today, I would have said, no. And I think most people in that area would have said the same. It is amazing the amount of progress not only in terms of science, but also engineering. If you look at the first chatbox, it is amazing amount of engineering that went in, but conversation is much more complex than question-answering which is a lot where we are right now. In conversation we can do a lot, we can manage different kinds of subjects at the same time, we stop one thread, we start another, we go back. Current systems cannot do that – that complexity in conversation. And we still have a lot to learn, possibly Large Language Models will be able to do that, although I am not sure, I have not seen much of that. When we come to things like I showed of getting that you have to saw a table and not the door, well, that has to do with common sense and intuitive physics which are things that we have not solved yet in AI. And I am not sure if neural networks will be the right technology to do that. We have not seen it solving that kind of stuff, they may solve it, but it might be that we just need different kind of architectures and structures to be able to do that. As someone who has been for thirty years in this, I have seen a lot of technologies coming and people saying “Oh, this will solve all the problems” and I think in the end they solve one big, difficult problem, like neural networks, they solve the similarity problem of finding things that we identify as similar that was very hard for computers to do. But not all problems are similarity, some involve common sense, some involve intuitive physics and that is going to take much longer. At the same time, what we have seen is that people when dealing with this, they learn how to be understood. Instead of asking Alexa, “What’s the weather for today?”, they say “Alexa, weather” because they learn they get the right answer. And that’s why I think it is getting to the middle and my hypothesis is that if Alexa speaks that way, in the way it actually understands, it may help people to do a language that makes sense and then we would have our language for machines that would evolve. There are a lot of papers saying that people are going into that direction, but it takes time.
Do you think that young children see AI as more human-like when having a conversation with it in relation to what you mentioned regarding the Turing test?
Children live in a world that is not exactly like ours, and that is the first thing that is important. If you have young children, they are still in the world where magical stuff exists, there is Santa Clause, there is magic. If there is a magic world, it does not need to be good; if there is Santa Clause, then also monsters exist, there is also bad stuff around. Children live in the world where things are too big for them most of the time. And perhaps AI creatures is one of those things, children live in a world they cannot explain, most of it. Children see agency much more than we do. Designing interfaces for children is extremely difficult, my only advice is test, test, test because most of the time children do not understand what you expect them to do.
Some time ago you presented a very interesting idea about villages where AI systems can be raised by community. Perhaps for those people who are not familiar with this idea you can briefly explain what it is based on. Also, I was wondering, do you think it would solve some of the problems that you mentioned and what shape would it have?
This is the idea from a paper that I didn’t show here today. The idea is that we should look into ways to develop chatbots, especially chatbots for more complex tasks, like an open source effort rather than them being developed by a company. And that has to do with the fact that knowledge is diverse. Suppose you are trying to create a chatbot to answer questions about some issue, say Sexually Transmitted Diseases, there is a lot of stuff, and perhaps the easiest way is to structure it as an open source that reflects the culture not of an individual but more of an area. I think we have had some success with things like Wikipedia and similar initiatives. So, I think it is something for us to test more as an approach, especially for chatbots that have to do with knowledge. It does not mean that there is one single truth, no, it is like Wikipedia, there are different opinions, but it has to be knowledge. It is interesting right now because I am starting to get involved into a project using AI to help with language issues, especially language documentation for indigenous peoples. And when it comes to that whole idea of a community developing the language of resources, it becomes even more important. But that is still very early, the project that we just started here.
A deep dive into the topic:
Pinhanez, C. (2019). Machine teaching by domain experts: towards more humane, inclusive, and intelligent machine learning systems. arXiv preprint arXiv:1908.08931. [pdf]