Josh Bongard: The Veinott Professor of Computer Science at the University of Vermont and Director of the Morphology, Evolution & Cognition Laboratory
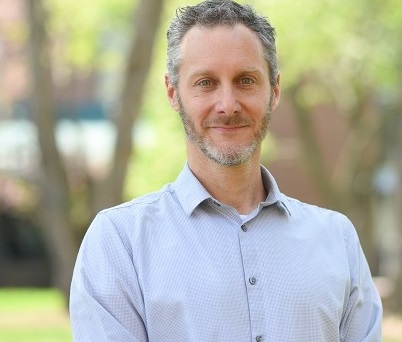
“He runs the Morphology, Evolution & Cognition Laboratory, whose work focuses on the role that morphology and evolution play in cognition.
In 2007, he was awarded a prestigious Microsoft Research New Faculty Fellowship and was named one of MIT Technology Review’s top 35 young innovators under 35. In 2010, he was awarded a Presidential Early Career Award for Scientists and Engineers (PECASE) by Barack Obama at a White House ceremony.
His work involves automated design and manufacture of soft-, evolved-, and crowdsourced robots, as well as computer-designed organisms. He is the co-author of the book “How The Body Shapes the Way We Think”, the instructor of Reddit-based evolutionary robotics Massive Open Online Course (MOOC), and director of the robotics outreach program Twitch Plays Robotics.”
Below is a transcript of the Q&A session
What would be the ways to study minimal cognition in these biobots, regarding an individual agent, and groups of agents? What could be the framework to do so?
Something that we are working on now is to automate the fabrication, which I mentioned earlier, so as to be able to 3D print or bio-fabricate large numbers of these xenobots and put them together individually or collectively in the dish. In addition, what we are looking into is building what is known as a robot scientist, basically, the ability to observe the behavior in the dish and record that as a video or a dataset and feed that observational data back into the genetic algorithm and close the loop. In the simplest sense, you could imagine a robot scientist that gives back one bit of information for each xenobot, which is whether or not it behaved like the simulation. From that the evolutionary algorithm can learn to avoid parts of the space of all possible simulated xenobots that do not transfer and focus more of the evolutionary effort on parts of the search that do seem to transfer to reality. That is sort of the simplest case. Once you have that loop closed, now you can really start to do things at scale; as you loop around from evolution to fabrication to analysis and back again, it gets easier and easier to take things from the evolutionary algorithm and instantiate them in the real world, and then you can add on top that loop questions about the nature of behavior. Not just which designs transfer to reality and which don’t, but why, what are some common morphological features or behavioral features among the ones that transfer well and those that don’t. The ones that don’t, it means that we’ve got something wrong about our model of xenobots, we’re missing something about the way cells communicate or the relationship between body and brain, we can start to really zero in on those kinds of questions.
Have you explored other materials? Things are being developed in material science, so apart from living cells, other materials can be used.
Yes, Professor Rebecca Kramer-Bottiglio runs mechanical engineering lab at Yale, and Rebecca and I have a funded collaboration to evolve what is known as metamaterials. Metamaterials are kind of interesting, they are very similar to xenobots, they act as traditional materials, but they also have other exotic properties, and they are very hard to design from basic engineering principles. So, in the same way that we evolve xenobots in silico and fabricate them in reality, we are now evolving metamaterials and Rebecca’s group at Yale is instantiating them as physical metamaterials. The step beyond that is obvious, which is to combine these two technologies. Biohybrid devices is another hot topic at the moment: how do you combine living and non-living materials to produce something that acts better than either alone. So, living components have their own advantages and disadvantages as do inert materials, can you combine them so that you get the best of both possible worlds. I think there’s going to be some really exciting things occurring at that interface in not-too-distant future.
What is a lifespan of a living organism and how can we extend it?
The xenobots that I showed you today, as I mentioned, they are taken from an early frog embryo and each cell has a little bit of egg yolk inside the cell and that’s like the battery. So, each cell has its own chemical battery, which provides enough energy for about 5 to 7 days. The moving xenobots that you saw, they’ll move about in a dish for about 5 or 7 days and then they will stop moving and then they’re just living material, they just rot away. You asked about how we can extend the lifetime; so the way we do that is to include other kinds of tissues, we are working on introducing other tissues, other ways for xenobots to metabolize external food so that they could start to metabolize or digest food in their environment and extend their lifetime in the same way that animals evolved to extend our lifetimes.
This AI field is fascinating and what would be the advice for those people who are interested in this field? Perhaps you could also tell a little bit of your story: how did you get into this field?
My undergraduate was in computer science, and I stumbled across a book that talked about evolutionary robotics, which seemed like a strange concept at the time. And what I showed you today is basically evolutionary robotics. The minute I started reading that book I realized I need to know more biology so as an undergraduate I tried to fit as many biology courses into my CS curriculum as I could which was not very many. And then for my master’s degree, I looked for master’s programs that were interdisciplinary, the master’s students were really forced to learn two fields at once. And I found a program at the University of Sussex which still exists today, this is the Evolutionary and Adaptive Systems, or EASy program, it is definitively not easy. So, for the undergraduates here, in Europe especially, it’s a great program, it’s been running for many years now, the computer science master’s students are forced into a wet lab and you have to build things from living tissue; the biology undergraduates that enter the program are forced to learn how to code, it is a coding bootcamp. So, both groups of students, the biologists and the computer scientists, they both suffer equally, they need to learn to code or learn how to manipulate living tissue. That was a big learning experience, and I think that helped me to really appreciate how difficult it is to work at the interface of two fields, but with perseverance, it showed me what’s possible if you persevere.
Where do you see this field going and what are you hoping for?
I think this is probably where Mike will start off next time, for now, I have been showing you xenobots as an engineering tool. There’s been a question in the chat what the applications might be. Hopefully, they will be able to help us with pulling plastics out of the ocean or scraping plaque out of human arteries, but that’s a number of years off. I think one of the potentials of xenobots moving forward is to help us with basic science. Imagine that we evolved a xenobot not to move quickly, but to be as conceptually transparent as possible, meaning that as the xenobot does whatever it does it’s advertising its function, it’s showing us how it figured out what to do, if you study naturally evolved organisms, for very good reason, they do not give up their secrets, they hide how they’re doing what they do for good evolutionary reasons. Can we evolve conceptually transparent organisms or machines? I think that is going to transform biology and artificial intelligence in the years to come.
A deep dive into the topic:
Bongard, J. (2014). Why morphology matters. The Horizons of Evolutionary Robotics, 6, 125-152. [pdf]
Gupta, A., Savarese, S., Ganguli, S., & Fei-Fei, L. (2021). Embodied Intelligence via Learning and Evolution. arXiv preprint arXiv:2102.02202. [link]
Roy, N., Posner, I., Barfoot, T., Beaudoin, P., Bengio, Y., Bohg, J., … & Van de Panne, M. (2021). From Machine Learning to Robotics: Challenges and Opportunities for Embodied Intelligence. arXiv preprint arXiv:2110.15245. [pdf]